Exciting News: Inflection AI acquires BoostKPI (Nov 2024)
Update: Meet the next-gen evolution: Inflection Insights (launched at Intel Vision, March 2025)
Kissht case study
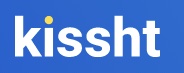
How Kissht uses BoostKPI to manage risk
Background and Problem
Kissht is one of India’s largest fintech companies, providing loans and credit to consumers. Data teams within Kissht were spending a lot of time determining the root cause of any changes in delinquency (loans that were not paid on time) and loan volume. It was critical for them to obtain quick business insights into the source of the changes. They wanted to do this without burdening their data team, which was working on more strategic data modeling challenges as well as ways to evaluate a prospective user’s credit score.
Kissht turned to BoostKPI to help them quickly and easily find the root causes of any changes in delinquency or loan volume. They wanted to leverage BoostKPI’s AI and ML capabilities to automatically slice and dice multi-dimensional, high cardinality data and determine the root cause behind any KPI changes.
BoostKPI setup
Kissht’s loan data was available in Snowflake. The data team then enriched that data based on other signals from the user’s profile. They then fed the data to BoostKPI, which enabled both on-demand root cause analysis as well as alerted them to any changes.
Kissht leveraged BoostKPI for multiple datasets:
Fresh users: Users who do not have any credit history and are fresh on the Kissht platform. The key dimensions are: binned loan amounts, binned credit scores, age bands, repayment risks, underwriting risks.
Users that have been on the Kissht platform for at least 3 cycles. Each credit cycle is just 28 days long. Every time a user, who typically did not have previous credit history, paid off their loan, they were progressively rewarded with more credit.
For their use cases, Kissht was not sure how to choose the driver variables. They wanted to iteratively define datasets. To better support their use case, the BoostKPI team added support for one-off imports.
The key KPI the Kissht team initially tracked was paid_T0_% – the percentage of users who were paying off their loans on time. With that, BoostKPI was able to point out the subset of data where big changes were happening.
Importance of picking a KPI aligned to business goals
As we mentioned earlier, the loan amounts for users kept on increasing in later cycles. In fact, the average loan amount in the 5th cycle was over 10x larger than the amount in the first cycle. Given the wide ranges of loan amounts, we found out that paid_T0_% was no longer a good KPI in capturing the business goal – the business goal was to see what % of the total amount loaned gets repaid, not just the count of loans that get repaid. Defaults on larger loans, though fewer, were more important to catch than defaults on smaller loans.
BoostKPI discovered and confirmed a curious phenomena. Users would repay their loans in earlier cycles. Once the loan amounts became significant, typically around the 4th or the 5th cycle, the users’ defaulted. The paid_T0_% was not a good KPI since it did not correctly align with this problem.
When the Kissht team updated the KPI to unpaid_%, BoostKPI was immediately able to highlight this root cause. A lot of the unpaid_% was coming from higher cycle counts.
Conclusion
Overall, the Kissht team has been pleased with the results of using BoostKPI. BoostKPI has helped them find root causes quickly and automate the manual slicing-and-dicing of data.
Amit Pingale, a lead data scientist at Kissht, says:
"BoostKPI allowed us to automate the manual anomaly detection of risk and volume by providing prompt alerts and root cause analysis."
Case studies